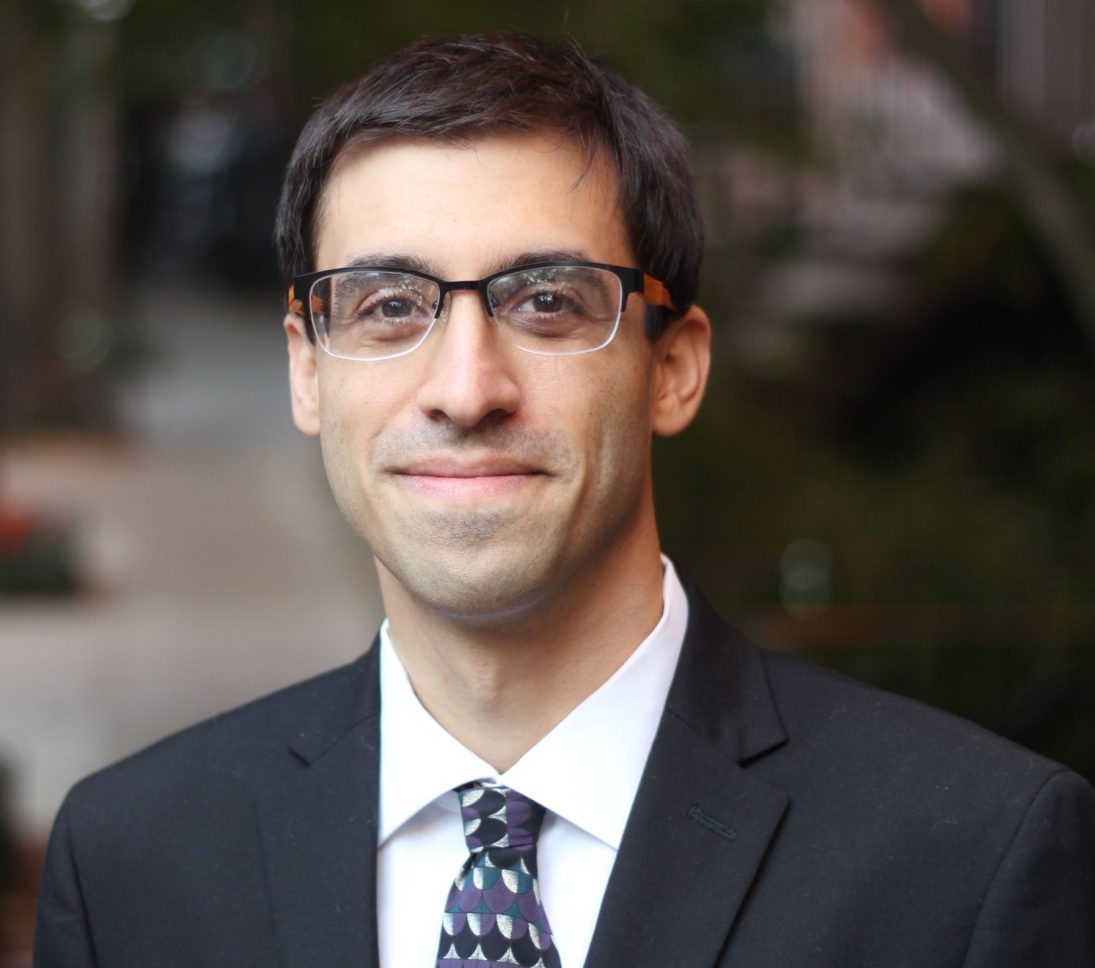
Sam Kapon [CV]
Assistant Professor, Berkeley Haas - BPP Group
Research Fields: Microeconomic theory, Experimental economics
Contact me at [skapon@berkeley.edu]
I am an assistant professor in the BPP group at the Haas School of Business, UC Berkeley.
Published and Accepted Papers
Dynamic Amnesty Programs [online appendix]
American Economic Review, 2022
Prior-Free Dynamic Allocation Under Limited Liability
with
Sylvain Chassang
Theoretical Economics, 2022
Using Divide and Conquer to Improve Tax Collection [supplementary materials]
with
Sylvain Chassang and Lucia Del Carpio,
Quarterly Journal of Economics, 2024
Working Papers
Persuading Cartelists to Confess
White Papers (Non-Research)
Designing Randomized Controlled Trials with External Validity in Mind
with
Sylvain Chassang